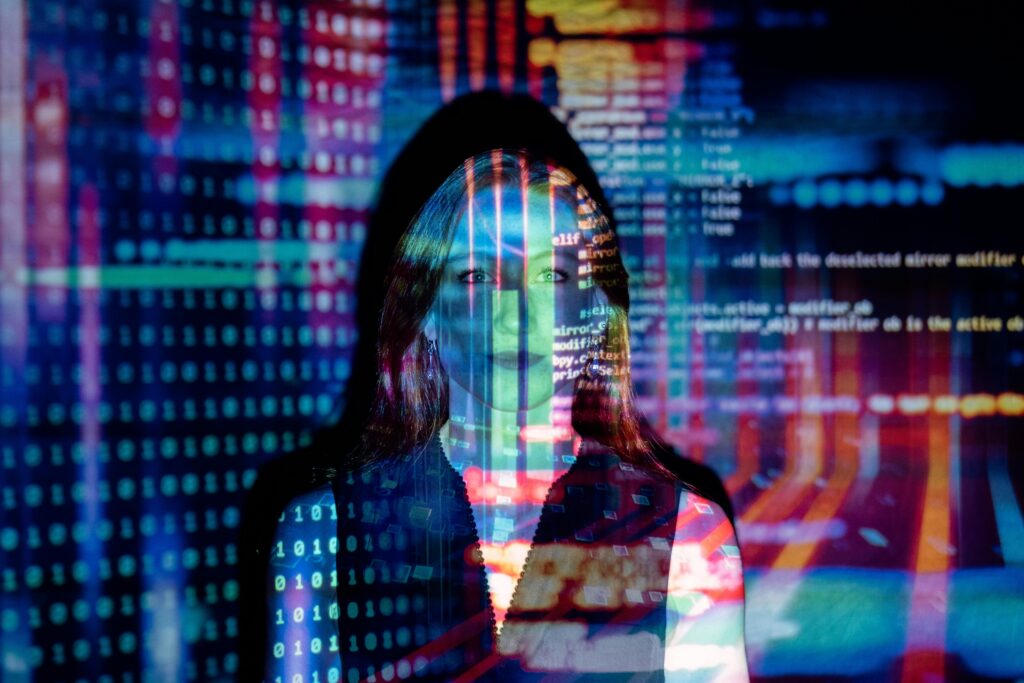
In today’s digital age, Artificial Intelligence (AI) and Machine Learning (ML) are two buzzwords that are frequently tossed around. Whether you’re scrolling through social media, using your smartphone, or even streaming your favorite show, chances are you’re experiencing these technologies in action. But what exactly do they mean, and how do they differ? In this article, we’ll dive deep into AI vs ML explained, highlighting their distinctions, applications, and future implications.
Table of Contents
What is AI ?
Artificial Intelligence refers to the simulation of human intelligence in machines that are programmed to think and learn like humans. The primary goal of AI is to create systems that can perform tasks that would typically require human intelligence. This includes things like reasoning, problem-solving, perception, and language understanding.
Types of Artificial Intelligence
AI can be broadly categorized into two types:
- Narrow AI: This type of AI is designed to perform a narrow task, such as facial recognition or internet searches. Most AI in use today falls into this category. For example, virtual assistants like Siri and Alexa are forms of narrow AI.
- General AI: This is a theoretical form of AI that would possess the ability to understand and reason about the world in the same way a human can. General AI remains largely hypothetical, as current technology has not yet achieved this level of intelligence.
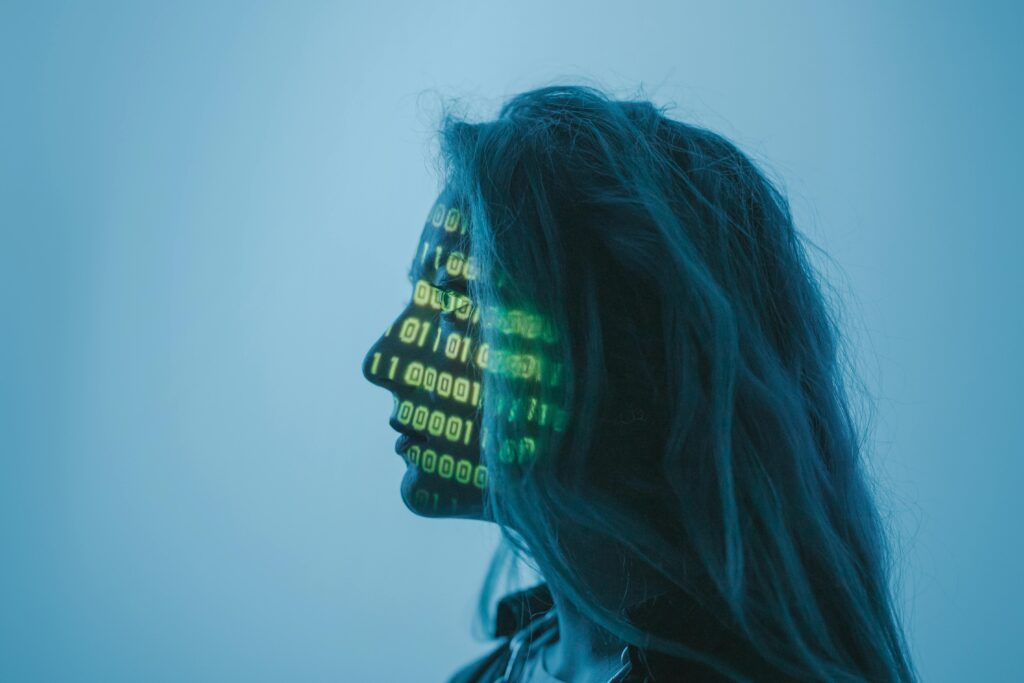
What is ML?
Machine Learning is a subset of AI that involves the use of algorithms and statistical models to enable machines to improve their performance on a specific task through experience. In other words, it’s about teaching machines to learn from data, identify patterns, and make decisions with minimal human intervention.
Types of Machine Learning
Machine learning can be divided into three main categories:
- Supervised Learning: In this type, the model is trained on a labeled dataset, meaning that each training example is paired with an output label. The algorithm learns to predict the output from the input data. Common applications include spam detection and image recognition.
- Unsupervised Learning: Here, the model works with unlabeled data and attempts to find patterns or groupings within the data. It’s often used for clustering and association problems, like market segmentation.
- Reinforcement Learning: This type of learning involves an agent that learns to make decisions by taking actions in an environment to maximize cumulative rewards. It’s widely used in robotics and game AI.
Key Differences BetweenAI vs ML explained.
Now that we have a foundational understanding of AI and ML, let’s explore the key differences between the two.
1. Definition
- AI: Encompasses a broader range of technologies that mimic human cognitive functions.
- ML: A specific approach within AI that focuses on learning from data.
2. Purpose
- AI: Aims to create intelligent agents that can perform tasks autonomously.
- ML: Seeks to improve task performance through learning from past experiences.
3. Methodology
- AI: Can utilize various approaches, including rule-based systems and algorithms.
- ML: Primarily relies on algorithms that allow systems to learn from and make predictions based on data.
4. Scope
- AI: Covers a wider scope, including robotics, natural language processing, and expert systems.
- ML: More focused, dealing primarily with data analysis and predictions.
5. Dependency
- AI: Does not always require data to function effectively; it can work on predefined rules.
- ML: Heavily dependent on large amounts of data to learn and improve.
Real-World Applications of AI
Artificial Intelligence has a vast array of applications across various industries:
1. Healthcare
AI systems can analyze medical data and assist in diagnosing diseases, predicting patient outcomes, and personalizing treatment plans.
2. Finance
In finance, AI algorithms are used for fraud detection, algorithmic trading, and personalized banking services.
3. Transportation
Self-driving cars are a prominent example of AI applications in the transportation sector, utilizing complex algorithms to navigate and make decisions on the road.
4. Customer Service
Chatbots powered by AI can handle customer inquiries, provide support, and improve user experience through quick and efficient responses.
Real-World Applications of Machine Learning
Machine Learning is also making significant strides across various sectors:
1. E-Commerce
Machine learning algorithms analyze user behavior to recommend products based on past purchases and browsing history, enhancing the shopping experience.
2. Marketing
ML helps marketers analyze customer data to segment audiences and personalize marketing campaigns, improving engagement and conversion rates.
3. Agriculture
Farmers use machine learning to predict crop yields, identify pests, and optimize farming practices, leading to increased productivity.
4. Cybersecurity
ML algorithms are employed to detect anomalies and potential threats in real time, helping organizations protect sensitive information.
Future of AI and ML
As technology continues to advance, the future of AI and ML looks promising. Here are some anticipated trends:
1. Enhanced Automation
We can expect to see even more automation in various industries, as AI and ML technologies become more sophisticated and capable of handling complex tasks without human intervention.
2. Greater Personalization
AI and ML will continue to refine their ability to personalize user experiences, from tailored recommendations to customized learning paths in education.
3. Ethical Considerations
As AI and ML become more integrated into our lives, ethical considerations surrounding privacy, bias, and accountability will take center stage, necessitating regulatory frameworks.
Conclusion
In summary, AI vs ML explained reveals that while both technologies share a common goal of enhancing capabilities and efficiencies, they are fundamentally different in their approaches and applications. Artificial Intelligence encompasses a broader spectrum of technologies aimed at mimicking human intelligence, while Machine Learning focuses on teaching machines to learn from data. As these technologies evolve, their impacts on various industries will only continue to grow, shaping the future of work, lifestyle, and beyond. Embracing these advancements while navigating their ethical implications will be crucial as we move forward in this AI-driven era.